The new article highlights the aspects related to the timeframes the applicants should follow.
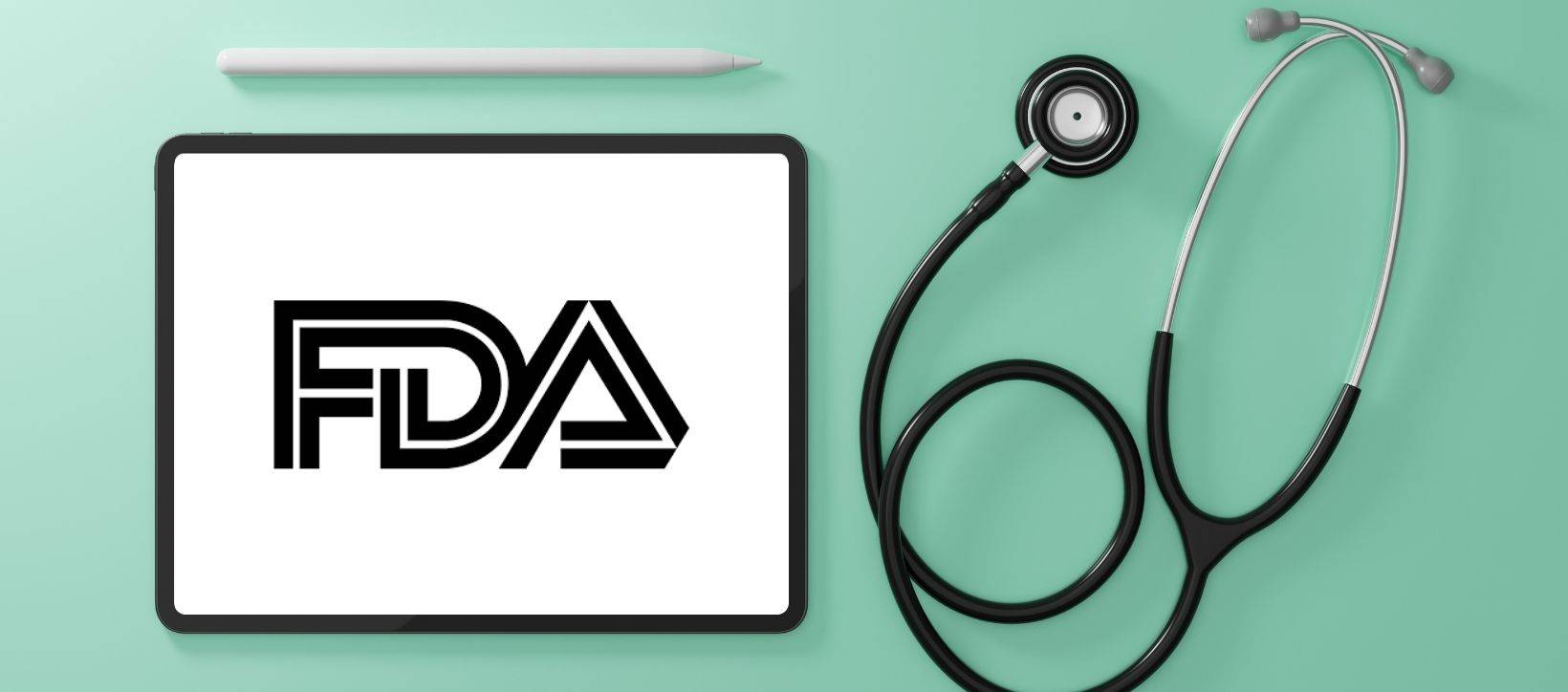
Table of content
The Food and Drug Administration the US regulating authority in the sphere of healthcare products, has published a guidance document dedicated to assessing the credibility of computational modeling and simulation in medical device submissions.
The document provides an overview of the applicable regulatory requirements, as well as additional clarifications and recommendations to be taken into consideration by medical device manufacturers and other parties involved to ensure compliance with that.
At the same time, provisions of the guidance are non-binding in their legal nature, nor are they intended to introduce new rules or impose new obligations.
Moreover, the authority explicitly states that an alternative approach could be applied, provided such an approach is in line with the existing legal framework and has been agreed with the authority in advance.
The document covers, inter alia, the aspects related to credibility evidence required to substantiate the claims made by the parties responsible for medical devices.
According to the guidance, computational modeling plays a crucial role in the development and validation of medical devices and patient-specific treatments.
These models, often sophisticated and complex, require rigorous validation to ensure accuracy and reliability.
Validation is a process encompassing various categories, each addressing different aspects of model credibility.
The present guidance describes in detail these categories, emphasizing their importance in medical device development and patient care.
In Vivo Validation Results (Category 4)
In vivo validation forms a basis of computational model credibility, focusing on patient-level comparisons using clinical or animal data.
As explained by the FDA, this validation is essential for patient-specific computational models, such as those used in clinical trials for medical devices. For instance, in fluid dynamics, a clinical software tool predicting Fractional Flow Reserve (FFR) in coronary arteries is validated against invasive clinical FFR measurements.
Similarly, bioheat transfer models predicting tissue heating from medical devices like ultrasound or radiofrequency energy are validated using direct temperature measurements in human or animal models.
Calculation verification and uncertainty quantification (UQ) play pivotal roles in these processes, ensuring the robustness of the validation results.
Population-Based Validation Results (Category 5)
Population-based validation contrasts with patient-level validation by focusing on broader population-level data comparisons.
This approach is particularly relevant for validating “virtual populations” or “virtual cohorts”, which are multiple patient models representing a patient population.
An example of this is in medical imaging, where virtual patient models are generated by varying parameters in an anthropomorphic model.
The computer-simulated trial results are statistically compared with clinical outcomes, ensuring that predictions align with actual human subject trials.
This category of validation underscores the importance of large-scale data analysis in verifying model predictions against comprehensive clinical datasets.
Emergent Model Behavior (Category 6)
According to the guidance, emergent model behaviour represents a unique validation approach, highlighting the capability of computational models to reproduce known phenomena under specific conditions, even when these phenomena are not explicitly modeled.
For example, in fluid dynamics, a model predicting blood flow through a stenotic vessel might be validated based on its ability to predict transitional or turbulent flow, a known phenomenon under certain conditions.
Similarly, in cardiac electrophysiology, models simulating electrical activity in the heart are validated based on their ability to reproduce electrocardiogram morphologies comparable to clinical ECGs.
This type of evidence underscores the model’s ability to replicate complex physiological behaviours, enhancing its credibility.
Model Plausibility (Category 7)
Model plausibility refers to the rationale supporting the choices of governing equations, model assumptions, and input parameters.
It is a form of evidence based on the theoretical soundness and justification of the model’s foundation.
An example is a finite element model of joint arthroplasty, where the plausibility evidence supports the credibility of the implant material model.
The model’s governing equations, assumptions, and input parameters are justified based on known mechanical behaviours and material properties, providing a strong basis for model credibility, especially when experimental data for validation is limited.
Calculation Verification/UQ Using COU Simulations (Category 8)
This category involves standalone calculation verification and UQ results performed using the Condition of Use (COU) simulations.
These simulations are crucial for in silico device testing or clinical trials where direct validation is not possible. For instance, a medical device’s finite element model might undergo a mesh convergence study to ensure minimal numerical error, supporting the model’s use in different COU scenarios.
In another case, a fluid dynamics model assessing changes in manufacturing tolerances on hemolysis in a ventricular assist device uses UQ to quantify the influence of geometric variances on predicted flow-induced stress and blood exposure time.
These examples illustrate the intricate processes involved in ensuring the accuracy and reliability of models under specific use conditions.
As explained by the authority, the various categories of validation evidence highlight the specific nature of computational model validation in the medical device sphere.
Each category, from in vivo validation to model plausibility, plays a crucial role in establishing and enhancing the credibility of computational models.
These models are vitally important in advancing medical device development, patient-specific treatments, and overall patient care.
As technology and medical science continue to evolve, so too must the methodologies and practices in computational model validation, ensuring that they remain robust, reliable, and relevant in an ever-changing landscape, considering rapid technology development.
Conclusion
In summary, the present FDA guidance provides a comprehensive overview of the various categories of validation evidence in computational modeling in the context of medical applications. The document outlines the essence of each category and its relevance in the broader context of medical device development and patient care.
How Can RegDesk Help?
RegDesk is a holistic Regulatory Information Management System that provides medical device and pharma companies with regulatory intelligence for over 120 markets worldwide. It can help you prepare and publish global applications, manage standards, run change assessments, and obtain real-time alerts on regulatory changes through a centralized platform. Our clients also have access to our network of over 4000 compliance experts worldwide to obtain verification on critical questions. Global expansion has never been this simple.